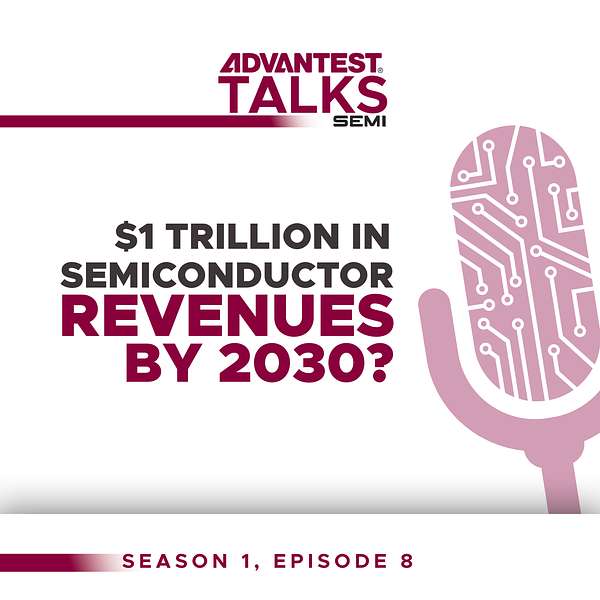
Advantest Talks Semi
Dive into the world of semiconductors and Automatic Test Equipment with our educational podcast, Advantest Talks Semi, where we explore the power of knowledge in this dynamic field. Hosted by Keith Schaub, Vice President of Technology and Strategy and Don Ong, Director and Head of Innovation for Advantest Field Service Business Group, at Advantest, this series features insightful conversations with experts and thought leaders in the industry.
In today's fast-paced environment, continuous learning is essential for staying ahead. Join us in these thought-provoking discussions, where you can learn about the latest trends and cutting-edge strategies being used in the semiconductor industry. Explore how innovative technologies are revolutionizing testing processes and shaping the future.
Stay updated on the ever-evolving semiconductor industry with Advantest Talks Semi, and gain exclusive insights into the future of technology.
The views, information, or opinions expressed during the Advantest Talks Semi series are solely those of the individuals interviewed and do not necessarily represent those of Advantest.
Advantest Talks Semi
$1 Trillion in Semiconductor Revenues by 2030?
Despite the pandemic, semiconductors hit a new global revenue milestone in 2021, reaching a record US$584 billion. Current forecasts project we’ll pass US$1 trillion by 2030. Is this realistic? With the test sector leading the way, AI and machine learning connected ecosystems are analyzing data in near real-time to gain new insights and help drive new advancements in IC technology.
Listen in as Keith Schaub, Advantest’s VP of technology and strategy, chats with Marc Hutner, senior director of product marketing, for proteanTecs, about some of the current thinking and innovative developments that can help us reach the $1T goal line.
Thanks for tuning in to "Advantest Talks Semi"!
If you enjoyed this episode, we'd love to hear from you! Please take a moment to leave a rating on Apple Podcast. Your feedback helps us improve and reach new listeners.
Don't forget to subscribe and share with your friends. We appreciate your support!
KEITH SCHAUB: Welcome to Advantest Talks Semi. Several industry sources have the semiconductor industry revenues growing to a staggering one trillion US dollars within the next decade, essentially doubling in size. Join Mark Hutner, Senior Director of Product Marketing at proteanTecs, and me as we discuss how AI and machine learning technologies being adopted across the semiconductor test ecosystem are essential to achieving the $1 trillion dollar target. Before we get started, here’s some important news from Hira. Hello Hira!
HIRA HASSAN: Hi Keith, I am very excited to give everyone updates on our annual VOICE conference. We will be hosting VOICE in person this year in Scottsdale, Arizona from May 17th to 18th. After hosting this event virtually last year, we can't wait to get back together with a bigger and better conference. Everything from amazing keynote speakers, technical presentations, sponsor booths, and of course raffles and prizes you won't want to miss. Our registration is now officially open so you can register at voice.advantest.com. We can't wait to see everyone under the hot Arizona sun, and as always, be sure to connect with Advantest on Twitter, Facebook, and LinkedIn for all the news and much more. Keith, that's the latest. Back to you.
KEITH SCHAUB: Thanks Hira. We're going to talk about semiconductor analytics, AI and machine learning, big data, all these new hot topics that are important in driving Era 4 the semiconductor industry. Era 3 was 585 billion last year and we’re slated to achieve one trillion mark in around 2031 or 2032. And the major portion of that growth is coming from AI and machine learning because if we look back 25 years ago, the focus was on the technology, making the measurement and the data was sort of an afterthought. The data was just something that was used to decide pass or fail. Now here we are, 30 years later, the data is king. The data is the most important and just want to understand your perspective on this, what are some of your experiences?
MARC HUTNER: Yeah, I think you're absolutely right in terms of your experience. You know, I joined the industry back in the late 90s where I was an instrument designer for test, and it was definitely make a good measurement and consider each test as a unique event. So how do I get to a spec limit? It was a real focus of the industry and now I think we're up to hundreds of thousands or maybe even as high as a million tests on a part. And now you're really getting into the how can I optimize that and look across tests? I think is a big trend that is really turned it on its head for our industry in general because I think that there is a lot more than the single spec. I think it's really the looking across specs and then doing an optimization has become very, very important. And if you kind of look back, you know, when we started this, we logged these things into text files and put them into simple databases. And then ultimately, in the 90s we moved into this domain of STDFs and binaries. And now we're even moving it one step forward with a couple of the new test standards where it's getting to two streaming test standards, like RITdb or TEMS, and it's really become like the immediacy of the data. Before we could get away with things like looking at the data after the whole lot was tested. Now we're really looking at as it's coming off the tester and making in situ decision. So, I think the way that we're thinking about data and add test is changing quite a bit.
KEITH SCHAUB: Yeah, that's some good insight Marc. I guess the key take away from me is that there's just tremendous value now there with the data. So somehow collecting this data because it's all valuable and utilizing it, we're just scratching the surface of what's possible.
MARC HUTNER: Yeah, and I think sort of those, the test techniques to collect the data has also been changing quite a bit. So, you know, if you kind of look back where we started, it was only possible to do that test on the tester and where we're moving is, you know, this common sort of test techniques can be applied at any stage, including the field. Now you've got this great possibility for correlation, which wasn't easy or possible before. It's very exciting.
KEITH SCHAUB: It feels to me that a lot of the data analytics and the data analysis that we're doing in industry, a lot of it is still very manual and so I suspect that it's quite tedious and difficult to extract the value. Have you experienced the same thing?
MARC HUTNER: Yeah, and I think that's the next part of the revolution that's going to occur is, how do you make it so that it's easy to come to some kind of conclusion or insight? You know, like with the instruments, each of these measurements are very, very specific to a situation. So how do you get that situational information and then apply it to multiple phases? So, we'll need to do that as an industry to make that a really getting value from the data itself will be a real big challenge for us.
KEITH SCHAUB: So, speaking of the value, what are some of the low hanging fruits that you see that maybe customers are already doing, but maybe some others that they're not doing that they could be doing?
MARC HUTNER: You know, in terms of low hanging fruits, it's really understanding what's changing on the die itself over its lifetime. So, where it started versus where it is and where it's going to be. I think it is really an interesting problem and you know, so for something like automotive or data center it will be vitally important. So how do I predict when to do a maintenance cycle? It's going to be extremely important because it ends up optimizing your maintenance costs of the vehicle or the data center. And I think that there's a lot of technologies that can be brought together including analytics to analyze the health. So, it does really start, you know, with reliability testing and you know, early ramp and characterization testing. But how do I build those models to really understand, you know, once I ramp a product how healthy it is over its lifetime.
KEITH SCHAUB: For the benefit of the audience that maybe is somewhat new to machine learning, talk about machine learning, how these models work, how prediction actually can come to pass and how it can be extremely accurate if the correct data and feature sets are chosen.
MARC HUTNER: Yeah, sure. The machine learning today is really based off of trained models. So it's how do I take a set of historical data and then ask the questions about, can I see a particular fault or a fault type and then what occurs as you kind of apply that data. So, you have to groom it a little bit to sort of have the right input features so you know why you took a particular kind of measurement and then you know, what do I want to learn from that data, and then you're passing it through basically a neural network. The idea is that you've created a model that has an understandable input output relationship. So the tricky part is really saying what are the input features that you want to consider to then lead to a prediction of an action. So that's actually where the bulk of the work is for a machine learning model.
KEITH SCHAUB: Like you said, selecting that feature set is really important and there are thousands of features, maybe tens of thousands of features. What Sony did is they figured out some feature sets from parametric test, and I bring that up because this is why it's so important to have the data ports or the data portals connected. So, they had some feature sets from parametric test data, and you can pair them or marry them with the test data during the in situ test and you can make some predictions. So, one of the things that was in the paper was with frequency test, and so you could actually predict what the result is going to be before you even made the measurement because you have access to this this data from parametric test. Right? So, I've predicted that I'm going to measure 10 and I measure 9.8, then I'm pretty sure that my measurement result is accurate. But if I predict that I'm going to measure 10 and I measure five, well something's gone awry. Either the measurement equipment needs to be recalibrated, maybe the process is drifting, or maybe my model is getting old and it needs to be retrained. But in all cases, something has gone awry and I want to know about it before I pass that device or fail that device.
MARC HUTNER: The tricky part is really what you want to learn. So, you know, proteanTecs itself has a lot of these kinds of parameters that we measure and one of them is a predicted leakage. We look at different process parameters that we can measure across the die and then do a prediction of what the aggregate on the die will do, that will end up saving a lot of time because it would have caused you to do a lot more kinds of measurements. So, there's a lot of things that we can do with a model but, I think the difference in how we're thinking about it is we start with what does the model need to do a certain kind of prediction, and then we're generating certain ondie features. And I think that's part of the next revolution is, how do you optimize the kinds of data that you are collecting? Because it's not an afterthought, it goes back to your, you know, data as an after thought versus an ML first or a prediction first mindset.
KEITH SCHAUB: One of the things that the industry has seen is the challenges of deployment. So, our industry is very much risk averse, basically Mark why is our industry so risk averse.
MARC HUTNER: Well, I think it comes back to the measurement thought of how do I predictably do something, right? I want to make sure I can guarantee a spec and anything about changing a setup, people get very nervous. So if I've been developing on a 93K for the last five years, I have a certain configuration, folks like to keep with that configuration because all their processes have to do with that setup in that mindset and that training of people. I think that even though folks are risk averse, they tend to find areas that you know, I'm developing the next 5G thing, and then they say stuff like there is no measurement technique to do it this way. So, it's really a where should I take risk and where does it make the most sense? But, we always tend to go back to tried and true methods, that's kind of what it comes back to is if it's possible to get you back to a known method, we tend to go there because we don't want to risk our product ramp against something that's unknown because there's enough moving parts. Like if you consider a 100,000 test numbers, you know, you don't want to make everyone risky, you only want the ones that really have to be risky, risky.
KEITH SCHAUB: Yeah, that's a good point and it reminded me of once we get something released into production, first of all, to get it released to production is a huge task, but once you get it released, you don't want to change it because if you do change it, you have to go through that entire process again.
MARC HUTNER: Yeah, and I think this is also where ML has a bit of a challenge, being accepted because it comes back to the explain ability of a decision. So, if you can have a method that changes limits around or gives a prediction, it's not only doing the prediction, I think it's vitally important to understand why it made a decision and how the model is changing if you're allowing for a real learning model. So, I think that there's several challenges in our industry of accepting this is a new technology, but I think that there's also a great opportunity associated with it. So, I think this is one area where we'll find that there are really thought leaders that are driving it, that see this as a huge opportunity. The rest of the market will follow suit once they have the proof point. Not sure if you read a really good book called the “Innovator's Dilemma,” but that one had a fantastic model of, you know, early adopters versus the midmarket, and then the late adopters.
KEITH SCHAUB: Well, speaking of early adopters, I was thinking about how to accelerate AI because again, we're talking about $500 billion growth over the next 10 years and every company wants their share of that. And so, the question I posed to customers and suppliers is, how are you going to get your share of that and really find the AI opportunities with the highest ROI? But, tomorrow's AI leaders are setting those strategies. They're organizing to start with low risk and high return pilot programs but, for the long term they have to have a strategy that aligns with the business strategy, and I get back to okay what are some of the easy, low hanging fruits? And I think the one that we talked about earlier where I'm predicting something and then verifying it, there's low risk in that, right? I'm making a prediction and then I'm just verifying my prediction, and that gives me more confidence in the result but, also gives me higher quality because now when I measure something let's say that's really good and it's not supposed to be really good, I'm not just going to pass that part, I'm going to perhaps bin it out. So, I think there are a lot of opportunities that credibility of the machine learning, you know the understand ability is not as important. It will be later but, I think there are a lot of opportunities where it's not so important just yet.
MARC HUTNER: I do think that there's several applications which will benefit from it significantly. The other one has been presented in a bunch of papers in terms of like pattern recognition for different kind of way for effects or tests running with geographic information. I think you can come to some very interesting conclusions with a little bit more knowledge versus considering a test in isolation. So, I think those ones are also apt for, you know, immediate deployment. I remember there was some papers from NVIDIA, where they map scan tests running on their devices and then they turned it into an image recognition problem. But it was very interesting to see how they were applying one set of problems against a known set of machine learning algorithms similar to, you know, how we're leveraging high power statistics. It's a, how do I form the data to use this algorithm to solve a problem? Primary reason for that kind of approach, and I think several kinds of problems are really well suited to that. And then I think that there's the next set of problems about what other data is really going to be needed to get to a better insight.
KEITH SCHAUB: You want to improve quality, right? So, if we want to get to autonomous driving vehicles, then we have to drive towards zero defects. We used to talk about parts per million, parts per billion, and now we are saying, no there can't be any. We want to get to zero. And the only way that you can at least approach that asymptomatically is by using machine learning, Let’s say we have all this data that's coming off of all of these testers and it's kind of funny, it's like we're looking for things that we know to look for and we find them. But, what we're not finding are the things that we don't know to look for. So it's the unknowns that where machine learning is really going to drive the value because let's say you have 10 important things that this data is telling you, maybe we're finding three of them. Now, these three things might be the most important ones, but as a company or as a manufacturer, I sure do want to know what the other seven are, especially if it's going to create a customer return or some sort of catastrophic failure in the future.
MARC HUTNER: I think what you're talking about is an ability for an institution to learn and get access to. It used to be fault models, right? So, we went from pretty much every semiconductor company that was doing digital, making their version of scan and ultimately there was the EDA industry that got formed that started to make tools and develop process to do stuff. I think machine learning, and it's kind of in that same spot back 20 years ago where the DA industry was, and it's really how do I get access to these more advanced relationships and learning of process technology to apologies. And then applying it back to chips and making it easy because, you know, your test and product engineers can't be an expert in everything. How do you quickly get to those insights becomes vitally important where it doesn't need a machine learnings expert? So, how can we kind of cross companies and get that learning? It really can be independent of process technology because I think the basic themes have been the same for these kinds of patterns. So, there's real possibility here.
KEITH SCHAUB: Let's talk about yield, so there's a lot of value and yield understanding and going back into the 90s, that wasn't generally the case. If I had to think about retrospectively why, I would say just we didn't have the volumes of data that were possible and now we do, we have. Once the mobility error hit and we were shipping billion phones a year, and now that data starts to be much more meaningful. Why is the yield understanding so important? Is it just because of there's so much data or are there other things that we should be thinking about?
MARC HUTNER: It used to be more of a black and white, this thing is working or not working. I think now there's more interfaces and blocks that require calibration and then the question becomes, can I recover a number of parts off of a wafer? And by the way, if you compare that against test time reduction, it's way more effective in terms of profitability to recover a percent of yield versus a percent of test time. So, it gets very interesting in terms of what does good really mean? And if you can continuously monitor that and make the perception from the customer that the product is working the same way, even with some tweaks in the background because that's what a lot of these interfaces are doing anyways, I think that's where the real value is. You can get more good parts, reduce your scrap costs. So how do I optimize that?
KEITH SCHAUB: I think what you're also saying is, a lot of these structures they build in after production calibration pieces that allow the product teams and let's say the manufacturing teams to tune that particular IP into the sweet zone, or into spec. That's another area where machine learning during the test actually could help do that. It can predict what those tuning parameters are, laser trim for example, is one of those where you want to trim something, but you don't want to over trim because once you do, it's too late.
MARC HUTNER: I think in the case of a laser trim, you're also trying to do a very coarse thing and then as you're in situ, you want to be able to sort of adjust for its aging. You get yourself into the neighborhood and then there's more softer pairs that can go on will become more and more important.
KEITH SCHAUB: So, let's talk about the technology employment of it. Are we ready to scale? I mean I see that this risk aversion and just the nature of our industry in the way that the supply chain is disaggregated and it's so complex, it's very hard to get, first of all, everyone to understand beyond that every customer is a little bit different. And getting all of that connected into some sort of infrastructure is no trivial task. But to me that's, that's got to happen if we want to really and truly take advantage of what we're talking about, you have to have that infrastructure in place to move data around, to protect it, to utilize it throughout the supply chain, and models have to be smarter than they are today. Like you said, most models today are supervised. Eventually someone has to come along and say, hey we need to update that model. That's going to have to change where the model is going to have to tell us when it needs to be updated, rather than we figure out that the model needs to be updated. So, there's a tremendous amount of activity going on in the industry.
MARC HUTNER: The good news is we're leveraging a lot of the pieces. It's not like we have to invent a new security model. We don't have to invent cloud computing. I think that there's a lot of base technologies throughout the industry that will enable us to get there. I think the other business motivation is, and this is talking to my own customers is, every one of them seems to have the goal of you need to be able to use machine learning at test because there's this value there. So, there's definitely a corporate motivation that if you're not doing it now, you're going to be missing out and you're going to not have an advantage in the future. So, I think the components are there, I think that the knowledge is there and it's really getting all of these pieces together. And I do think from a data transmission standard point of view, it's also there. So, I think we've got the right components now, and it's really a question of the companies that figure out how to combine these components and who to partner with will get an advantage over the next couple of years.
KEITH SCHAUB: So, you talked about your company proteanTecs. proteanTecs is an Israeli based company that deals with machine learning and AI, so give us the background on the company and why is it different from others in this category?
MARC HUTNER: We are a data analytics machine learning company that's really focused on deep data for health and performance of systems. So, we're really trying to figure out how to get the most performance on your systems and how to, whether they're starting to age or whether you can tweak out that little bit of performance. Now, we were formed about in 2017. There was three founders of Mellanox formed this company, and it's really addressing problems at industry scale. So, we're able to scale up using cloud computing and we can also scale down using edge computing at a tester. Now we're silicon proven multiple process nodes already even though we're a five-year-old company, and our primary markets are really data center, automotive, and recently announced communications. But, what we've discovered through our product is really you need a multidisciplinary approach. So, we have a lot of machine learning experts but, we also start all of this with design features. So, we kind of have to combine all of those skills. So, I should probably mention a little bit about what I mean by, “deep data.” What it really comes back to is, what are the process and technology features that are needed to understand the health of a system or a piece of silicon. So we've developed something called U. C. T. (Universal Chip Telemetry), and that combined with focused machine learning algorithms, has enabled us to drive new insights and so as I mentioned the models can run on edge and cloud, but it's really the combination of those three things that the deep data generated from our U. C. T, the machine learning algorithms, as well as the cloud or edge computing, all combining to give you this super understanding of the health and performance of your system. The starting point is that there are several kinds of I. P. that we can insert into your design. It's a combination of hard and soft I. P. So, we do a lot of simulations at the front end of the design process. And one example of a U. C. T. agent is really our margin agent. So, what we do is we look at all of your timing runs and then we have a way to identify the critical path. We give recommendations on those and then we insert the soft I.P. that will say how much margin do you have on each of those? Now, what you can do with that gets kind of interesting, which is I can run it wafer tests but, I can also run it in the field. So, I can keep track of it in the field, oh I had 30 seconds margin, oh it went down to 20 seconds. With that agent, and using machine learning, and using a reliability study, you can then predict at what point you'll have a failure. Now, we have all sorts of different kinds of agents. We have operational ones, we have ones on Interconnect, ones that are for classification and profiling of process and technology. So, there's lots of different kinds that we do insert into people's designs, and then it's really having that library of algorithms that you can run on top of those measurements. So, and our algorithms are really looking at multiple parameters as part of it.
KEITH SCHAUB: Okay, so let's talk a little bit about the aging specifically for autonomous vehicles. When you think about autonomous vehicles, it's extremely important that these chips inside the vehicles actually can communicate to the vendors, to the suppliers, about when they need maintenance or when there could potentially be a fault. If that's happening with our laptop, that's one thing, it crashes. No big deal, we buy a new laptop but, if that's happening with an automobile and there's potentially a fatality or a catastrophic event, proteanTecs is working on some fascinating things there. What can you say about that?
MARC HUTNER: Yeah, so the amount of content in the car has been increasing considerably. If I look at the car, I have now versus the car I had, just over 10 years ago, you know, the number of microcontrollers and the amount of processing of data has exploded. And you know, from a user perspective, it's very exciting. You know, I can do things like see the car go beside me and then I get a little warning light. But on the other hand, it does add a lot of complexity and car companies are just getting used to this level of complexity. I'll tell you a story, from about 10 years ago, where a board went bad on my hybrid and they just kept swapping boards on that car until they found the right one. So having the ability to say that this chip for the subsystem is going wrong is extremely valuable, and one of the technologies that we offer is really understanding the health of a system like that. So, we'll be able to identify not only that a subsystem has gone bad but, where in that chip could be an aging or reliability problem going forward, and the really cool part is it could get you to predictive maintenance. So, we'll be able to see sort of how that device is aging over time and so that way you can bring it into the shop and basically get that component replaced.
KEITH SCHAUB: For today I think that's all we have time for. I want to say thank you for coming on the show and sharing your insights and experience with us and looking forward to some future updates from you and proteanTecs.
MARC HUTNER: Thank you, Keith.
KEITH SCHAUB: To round out this week's episode, here's Junko’s top three. Hi Junko.
JUNKO NAKAYA: Hi Keith. So, I'm sure you are noticing this too, but in person events are slowly making a comeback. In fact, Advantest exhibited at SEMICON West and SEMICON Japan last December. So, what I wanted to tell you today is how these shows went. So, here we go. My first takeaway is about SEMICON West 2021, which was held in San Francisco in December, the show traffic was much lower than previous years as expected but, customers who came to see us was so very happy to see us and very happy to have face to face conversations with us. In addition to the exhibition, Advantest presented multiple papers and moderated a panel discussion at Test Vision Symposium during SEMICON West. So, we were very busy. Then, my second takeaway is about SEMICON Japan, which was right after SEMICON West. While the overall show attendance was down by about 50%, Advantest booth welcomed as many customers as previous years. Then, again, our customers are so very happy to see us and learn about the latest test solutions. Speaking of the latest test solutions, my last takeaway is that we successfully showcased our latest test solutions at both SEMICON West and SEMICON Japan, including some new products like B93000 links scale, T5385 memory platform, T2000 I. P Engine 4 and ACS, all of which support our mid and long term strategy, Advantest Grand Design. So, I hope you continue to see us more at in person events this year. As mentioned earlier in the news section, we will be in Scottsdale, Arizona in May for VOICE and we will be at SEMICON China in June, just to name a few. So, I hope the listeners of Advantest Talks Semi Podcast series will come and visit us. Back to you, Keith.
KEITH SCHAUB: Thank you, Junko. Well that does it for another episode of Advantest Talks Semi. See you next time.