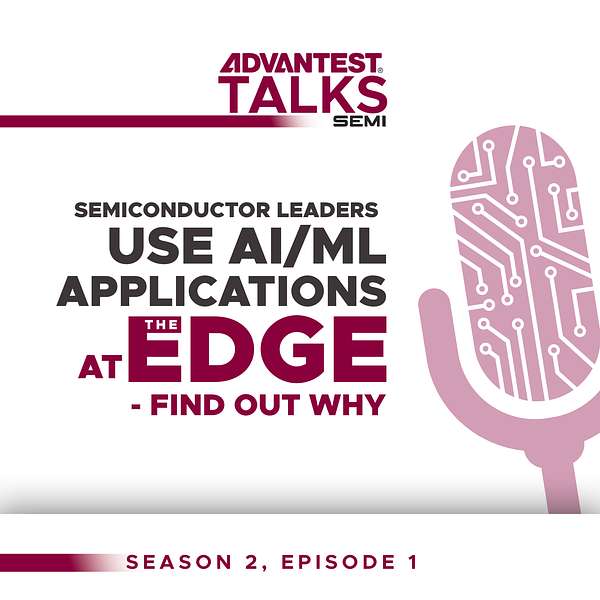
Advantest Talks Semi
Dive into the world of semiconductors and Automatic Test Equipment with our educational podcast, Advantest Talks Semi, where we explore the power of knowledge in this dynamic field. Hosted by Keith Schaub, Vice President of Technology and Strategy and Don Ong, Director and Head of Innovation for Advantest Field Service Business Group, at Advantest, this series features insightful conversations with experts and thought leaders in the industry.
In today's fast-paced environment, continuous learning is essential for staying ahead. Join us in these thought-provoking discussions, where you can learn about the latest trends and cutting-edge strategies being used in the semiconductor industry. Explore how innovative technologies are revolutionizing testing processes and shaping the future.
Stay updated on the ever-evolving semiconductor industry with Advantest Talks Semi, and gain exclusive insights into the future of technology.
The views, information, or opinions expressed during the Advantest Talks Semi series are solely those of the individuals interviewed and do not necessarily represent those of Advantest.
Advantest Talks Semi
Semiconductor Leaders use AI/ML Applications at the Edge - Find Out Why.
Artificial intelligence has significant value-creation potential in the semiconductor industry with recent value estimates in the mobile industry alone in the $100’s of Millions to over $1 Billion dollars. To capture this value, leading semiconductor device manufacturers want to unleash and scale their proprietary AI and ML methodologies in the intensely competitive winner-takes-all environment where aggressive innovation is required while product life cycles continue to shrink.
Listen in as experts from PDF Solutions, Vishnu Rajan engagement director, Greg Prewitt director of product management, Excensio test operations, and David Park, vice president of marketing, join Advantest Talks Semi and learn the latest of what is happening at the “edge” of semiconductor test.
Thanks for tuning in to "Advantest Talks Semi"!
If you enjoyed this episode, we'd love to hear from you! Please take a moment to leave a rating on Apple Podcast. Your feedback helps us improve and reach new listeners.
Don't forget to subscribe and share with your friends. We appreciate your support!
KEITH SCHAUB: Artificial intelligence has significant value creation potential in the semiconductor industry. For the mobile industry alone, Advantest has estimated the value in the hundreds of millions to over $1 billion. How can semiconductor companies deploy AI and machine learning at scale to capture this value? A recent survey reported that only about 30% are generating value through AI and ML, and it's important to note that these companies have made significant investments in AI talent, in infrastructure and technology, and they have scaled or are scaling up their initial use cases. The other 70% are still in pilot phase and their progress has stalled. I believe that the application of AI and ML will dramatically accelerate across the semiconductor test value chain over the next few years. So the question becomes, how can semiconductor device manufacturers unleash and scale their proprietary AI and ML methodologies? They need to do this in a winner takes all environment where product life cycles continue to shrink and aggressive innovation is required to stay competitive. Hello and welcome to Season 2 of Advantest Talks Semi. I'm your host, Keith Schaub, Vice President of Technology and Strategy at Advantest, and to answer these challenging questions, I'm joined by experts from PDF Solutions, Vishnu Rajan, Engagement Director; Greg Prewitt, Director of Product Management Exensio Test Operations; and David Park, Vice President of Marketing. Vishnu, David, Greg, welcome to Advantest Talks Semi.
DAVID PARK: Thanks for having us, Keith.
GREG PREWITT: Glad to be here. Thanks for the invite.
VISHNU RAJAN: Yes, thank you very much. Happy to be here today.
KEITH SCHAUB: Yeah, great guys, thanks for coming on. Hey, in the opening remarks, we highlighted the constant competitive pressures of the semiconductor industry. It's really a brutal industry and the stakes are getting increasingly high. So for example, the design costs have increased from around $28 million at the 65 nanometer process node, which feels like ages ago and now it's over half a billion dollars at the leading edge five nanometer node. Combine that, that at the same time we've got FAB costs increasing more than 10 x going from $400 million to over five billion. It's really an understatement to say that this is getting pretty expensive. All that investment has to get tested and the industry is demanding innovations, especially in production testing where cost, quality and yield are paramount. So I'd like to start our discussion on the edge, because billions of parts per year get tested on Advantest systems like the V93000. So there's a lot of emphasis on edge applications. Can you walk us through why edge applications are seeing this emphasis?
DAVID PARK: Sure, Keith. I think there are two things that are driving the interest in edge apps. One is the point you brought up which is that there are billions of devices being tested. It's probably closer to trillions but I don't know the exact number. But those billions of devices are generating terabytes and terabytes of data and it's very time consuming and costly to have to move that data from the tester and from the test floor to some centralized point where people can analyze the data, make a decision and then turn that decision into an action back on those testers. And that leads into the second point which is that time delayed to take that action can be costly. Product is continually flowing through. They don't stop the test floor while you decide whether or not you're going to make a decision. Products are continuing to be tested all the time. So the quicker you can turn around that data and make a decision, the better off your overall test operations are going to be. So that combination of the volume of data and the desire to have a more speedy action or decision being taken based on what the data is showing I think is what's pushing things out to the edge.
KEITH SCHAUB: Vishnu, what are your thoughts?
VISHNU RAJAN: Thank you, Keith. I would add also that being able to make a decision, or I'll even use the phrase insert a decision, either at the point of data collection or very close to the point of data collection also has the benefit of, well not only are you making that decision in real time, you are also adding to the data that you're collecting and enabling that for further downstream usage. You're augmenting and you're supplementing and now you're going beyond just what you've collected. That in itself can also prove to be very valuable by enabling further downstream applications.
KEITH SCHAUB: So Greg, what do you think?
GREG PREWITT: I agree with your assessment of, you know, the cost of devices and the complexity and it's the test operation of these complex devices is resulting in massive amounts of data. And further, as you mentioned, data does have a shelf life, and it's most actionable at the time that the data is generated. That makes for an ideal application for machine learning, running in real time and at the edge, giving you the capability to influence the current test operation and, you know, add value and make decisions for subsequent test operations that will follow.
KEITH SCHAUB: Going back to what you said, David with terabytes of data. So as customers take advantage of big test data and make more and more decisions at the edge where the decision is most valuable and timely, then security becomes another critical issue for customers, and our industry is surrounded by geopolitical tensions, IP concerns, counterfeiting concerns. We've got ransomware, hacking, you name it. And as we previously mentioned, this industry is brutally competitive with a winner take all, or at least a winner take most, environment. The successful companies that are moving towards analytics at the edge, they are also establishing a connectivity layer for real time access of the data sources. But more than that, they are demanding this zero-trust security mindset and that needs to be an integral part of the architecture. Without that built in mindset and architecture, it's essentially a nonstarter. And what I mean by that is the security can't be an add-on or it can't be an afterthought. Security must be deeply rooted in the core. How do you see this impacting the adoption of cloud and edge solutions, and what do you think are the top security concerns, and how are customers mitigating risks, but also taking advantages of the opportunities?
GREG PREWITT: Customers are showing concern for their test data in their discussions with us, and they use terms like zero-trust. Customer base has evolved over the years. They used to not want to consider cloud-based systems. They were always keen on having their applications run and hosted locally, but that perception has changed drastically in the last four years or so. Cloud systems have proven themselves as a viable alternative with probably higher security levels than you know they were getting from their in-house solutions. And while the concept of zero trust is appealing, in practice I see it a limited trust model is being more practical. For instance, Advantest has recently implemented encrypted data logs and SmarTest versions which gives the customer control over their data. To be able to implement test process control and monitoring, this data must be decrypted at least for a point in time. And then resealed to maintain the confidentiality of that data. The use of containers allows the customer to encapsulate their machine learning models or algorithms into a trusted execution environment where the customer can minimize the contents and vet the contents of that execution environment. Other blocks of data, however, may be allowed to flow through the test ecosystem completely encrypted from the source at the tester and remain totally confidential until it reaches the customer in point. And this degree of control can be accomplished by the management encryption and decryption keys so that portions of the data stream can be made available for real time decisions in processing and control, and yet other parts of the data remains confidential until it reaches their system.
KEITH SCHAUB: And David, what about the security standards? What's going on there?
DAVID PARK: Building on what Greg just said, there is also a broader industry need for security and actually, there is an effort ongoing that's being driven by the Global Semiconductor Association, or the GSA, the initiative is called TIES, or Trusted IoT Ecosystem Security. And the concept behind this is that we have to share data today. It may not be done elegantly. It may not be done easily or efficiently, but data is being shared and the idea is to do it in a way that makes it much more convenient for not just the semiconductor industry but the much broader electronics industry as well as upstream participants such as IP providers and to make the data flow more seamlessly through the entire supply chain. So the idea behind it is to build a trusted collaborative ecosystem that makes sure that people who are generating data, that only trusted people have access to that data, and the data can flow all the way through even to people who may see semiconductors as a black box. And actually both PDF Solutions and Advantest are both members of the working groups of GSA TIES. And for those people who are listening to this podcast, I'd encourage you to go to the GSA Website, it's actually GSAGlobal.org and look at their working groups and look for the TIES working group. And perhaps you might be interested in either joining or being a contributor to this effort because it has a very significant number of major players in the industry, not just semiconductor providers, but OSATs, electronics companies and system companies and everyone is very keenly interested in how the overall industry can make data sharing more secure, but also more seamless and easy to access.
KEITH SCHAUB: Okay, so we've talked about the emphasis of edge-based applications and that brought with it a bunch of security concerns and there's a lot happening in the industry around that to enable edge applications at scale. Now let's shift over to the value of edge-based applications. The sort of value that customers are seeing. Manufacturing is the semiconductor industry's largest cost driver. Hands down, manufacturing will accrue the most value from advanced analytics, which is not a surprise given the expenditures involved in semiconductor fabrication and I've seen estimates that AI and ML are expected to deliver up to around 40% of the overall value here by reducing costs or improving yields and improving efficiencies, just to name a few. So that’s hundreds of millions or over a billion dollars, 40% of that. Most of that's going in for manufacturing. Given that customers want to do analytics at the edge and the industry is getting more mature for how to provide security and trust to perform those analytics, rather than just doing it within their four walls, what sort of edge applications are customers deploying to reduce the cost or to improve the yield or improve efficiencies?
VISHNU RAJAN: Thank you, Keith. It's a great question. Starting kind of at the higher level. The business metrics of interest are the ones that you highlighted: cost of test, quality and escape prevention, and then operational efficiency and throughput optimization. The umbrella, all that falls underneath, is what we talked about previously around security and so forth. Getting into each of those business metrics, there are different applications that we see customer interest for, outlier screening in particular. Being able to do this in real time at package test, or as some people refer to it, final test. The advantage of doing it in real time at the edge as the test is completing, you eliminate the need for a post process step. The part gets tested, you detect right away, “Hey, is this an outlier or not?” You can disposition it without any additional post processing that's needed. This is of interest for customers. In a related vein tester process control, and what we mean by this is applying SPC WECO rules to the tester process itself. So for example, consecutive bin. Hey, I'm getting the same bin over and over again. Something’s probably not right here. I better pause or stop my test as soon as I can because I want to prevent some escapes. Site to site yield. Hey, maybe I see a big difference here. Something maybe isn't quite right and I need to have an operator intervene and check and make sure the test set up is correct. These kinds of things: outlier screening, test or process control. These are of high interest in terms of quality and escape prevention. Moving to the area of operational efficiency, what we see customers wanting is how to balance their available test capacity without going quality. So, in other words, what's the best way that I can apply the test budget I have so that I maximize my die out without sacrificing quality? And in this regard, what we see interest for is adaptive test. So this is the ability to dynamically decide, do I want to test more or do I want to test less? In the test less example, you have a stable baseline and then you decide, “Hey, you know what, this lot is running really good. I'm going to reduce the number of tests on each die through something that's prescribed and planned and so that way I can get more die out with my test capacity.” The reverse would be to say, “Hey, I have a very sensitive part. Maybe it's a medical or an automotive device and hey, I see some instability. Something doesn't look quite right here. You know what, I'm going to turn on some additional tests because I really need to make sure these parts are good.” And the last piece, which is somewhat of a slightly newer concept is the idea of doing real time re-binning. We refer to this as statistical binning and the concept here is, I'm going to monitor a whole suite of tests. Maybe pick a number 10, 15 could be some number of tests and I'm going to watch those tests and based on the results of those tests, I'm then going to decide should I re-bin this device. So an example here would be something like, all 10 tests I am watching formally speaking, they're all passing, they’re within the limits. But, you know, they're all like two sigma three sigma. They're kind of pushing the upper bound of what I'm comfortable with. So you know what for this device, because all these 10 tests are in that upper range, you know what I'm gonna down bin it because I want this device to get some additional, maybe it's burn in some additional testing at package test and so forth. These four applications. These are the kinds of things that we see customer interest for.
KEITH SCHAUB: Well that's great, Vishnu. Thanks for your insights into these applications. Before we wrap things up, and you know just looking ahead, what can the industry look forward to on the horizon for edge and cloud and other types of analytics? David, you want to start with that?
DAVID PARK: Absolutely, Keith. I think as we've seen in the prior parts of the podcast, the use of edge analytics is not only going to continue, it's going to accelerate. And the most logical progression is that not only will customers be doing analytics at the edge or statistical based analytics, they're going to start doing a lot more AI and ML. But it's not going to be a simple step because machine learning applications and AI need to be able to take into account or be adaptive to what happens, and I think we're getting there, but as we've seen for things like autonomous driving in cars. It's pretty good, but it's not perfect, and usually when it's not perfect some unfortunate incidents happen, but it's definitely gonna happen because the value is there for customers. They know the value of time to data and time to action. And I think as Greg mentioned earlier, we're also getting to the point where it's just something not practical for human beings to be able to analyze the volumes of data that are being collected, so you need to apply AI and ML just to consume and go through all the data that's being collected and stored and saved. But I think there's a lot of opportunities for optimization and hopefully when you invite us back for another podcast, we might have some interesting news to share with the audience.
KEITH SCHAUB: Definitely, David. So hey, Vishnu, your thoughts on that? What's coming or what do you see out on the horizon?
VISHNU RAJAN: Great question, Keith. One of the things, you know, as we’re talking through where we see current customer interest, a lot of the things I mentioned are statistically based, you know, we're applying known statistical decisions to then, you know, produce a result. Where we see that changing in the future is shifting those decisions to be machine learning based, applying artificial intelligence to make those decisions, and the kinds of benefits that we can expect to see from that is to say for example, capturing more outliers, but minimizing your amount of overkill the more you can dial in a model to do that, that’s a good thing, and that's where really where we see customers wanting to go. Hey, only very selectively, let's kill out the bad die. Let me keep all my good die.
KEITH SCHAUB: Great. And Greg, you want to close it out for us? What do you see?
GREG PREWITT: Yes, Keith. Our customer base has shown a clear interest in machine learning at multiple steps throughout their operation. And I think that there's a couple of basic reasons for that. One is in our own work. We have proven that the machine learning models can be more selective, in other words, have less overkill, than outlier detection algorithms based on classical statistics. So they're more selective. You're not going to have as much good devices tossed out to catch the marginal devices. The other is the customer base is clearly asking questions, have done some amount of implementation of data feed forward, so they're using machine learning models to make predictions about die. They're doing that typically today between test operations but then need to implement these die-grading scores at a test operation and then the next frontier is clearly going to be to make these predictions in real time affect test operation that's in process right now with the die in situ and that's why we're excited to work with Advantest on the ACS edge. It gives that kind of compute power in a real time domain.
KEITH SCHAUB: Okay, well that's fantastic and super exciting. I don't know about you, but I see this as truly an amazing journey that entire industry is on and you guys have given us some insightful aspects of what's coming, what already exists and what's possible and where things are headed and to me it’s all very fascinating. So I’d like to thank you Vishnu, David and Greg for taking the time to Talk Semi with Advantest.
GREGG PREWITT: Thank you. Glad to be here.
DAVID PARK: Yep, thanks for having us, Keith.
VISHNU RAJAN: Thank you very much. This was great and look forward to doing this in the future.
KEITH SCHAUB: Thank you guys and that does it for another episode of Advantest Talks Semi. See you next time.
KEITH SCHAUB: Welcome to our Advantest Talks Semi post-show segment. If you've enjoyed the podcast thus far and are wanting more, then you've come to the right place. Continue listening in as we go deeper into some of the engineering and technical discussions. So Greg mentioned that machine learning could be more selective than or has a higher selectivity with the outlier control and I was wondering why that is. Do we have some thoughts or insights as to what makes it have a higher selectivity?
GREG PREWITT: Most of the standard outlier detection algorithms that are based in classical statistics are based on some form of variation. In other words, standard deviation, either between adjacent devices or between the population of devices, where the machine learning models take in many more parameters. So instead of being kind of a uni-variant model, they’re multi-variant in their nature. They can consider hundreds of parameters practically. And also, the machine learning models tend to be trained against a specific historic data set. That makes them more selective. The only gotcha with that is then you need kind of a workflow to produce these machine learning models where you have historic data, you recall that historic data, you've labeled that historic data. Therefore, you can train on the historic data and produce a model, then it's well tuned and effective for future production.
KEITH SCHAUB: And that does it for another episode of Advantest Talks Semi. See you next time.