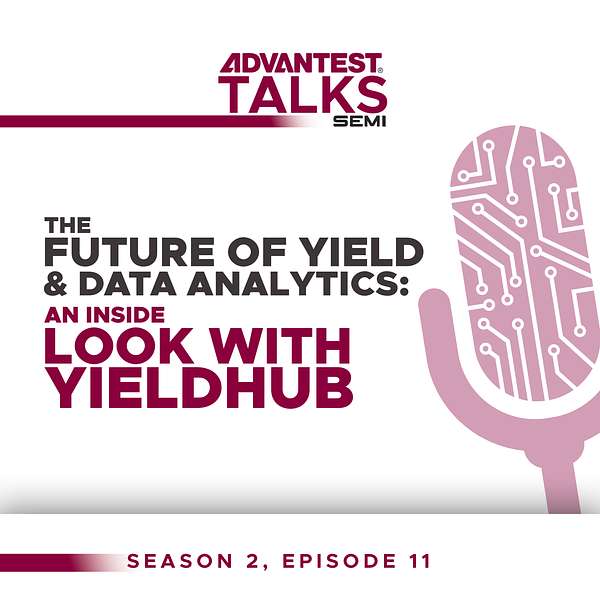
Advantest Talks Semi
Dive into the world of semiconductors and Automatic Test Equipment with our educational podcast, Advantest Talks Semi, where we explore the power of knowledge in this dynamic field. Hosted by Keith Schaub, Vice President of Technology and Strategy and Don Ong, Director and Head of Innovation for Advantest Field Service Business Group, at Advantest, this series features insightful conversations with experts and thought leaders in the industry.
In today's fast-paced environment, continuous learning is essential for staying ahead. Join us in these thought-provoking discussions, where you can learn about the latest trends and cutting-edge strategies being used in the semiconductor industry. Explore how innovative technologies are revolutionizing testing processes and shaping the future.
Stay updated on the ever-evolving semiconductor industry with Advantest Talks Semi, and gain exclusive insights into the future of technology.
The views, information, or opinions expressed during the Advantest Talks Semi series are solely those of the individuals interviewed and do not necessarily represent those of Advantest.
Advantest Talks Semi
The Future of Yield and Data Analytics: An Inside Look with yieldHUB
John has a degree in Microelectronics from UCC (University College Cork) and spent more than 17 years at a leading semiconductor company before starting yieldHUB (formerly MFG Vision). Fast forward to today and he's running a company with a platform that's used by over a thousand of product users around the world. yieldHUB exists to help semiconductor customers maximize their yield. John loves what he does and enjoys traveling the world to meet new prospects and customers.
Carl is a semiconductor and yield management expert with a career spanning 40+ years. During his dynamic career, Carl’s held technical management positions across product and test engineering, assembly, manufacturing, and design at established semiconductor companies. Carl is passionate about data analytics and has a reputation for building strong customer relationships.
Data is the lifeblood of the semiconductor industry, yet managing new data sources and formats is a colossal task. With our guests, we'll explore how yieldHUB's platform streamlines data integration, and how artificial intelligence (AI) and machine learning are shaking up production testing. We'll also discuss the merger of human expertise and AI to achieve optimal results and the global scalability of data analytics through cloud-based solutions. You’ll be awestruck by the sheer potential and power of these technologies.
John and Carl will share their insights about the unique features of yieldHUB, including its ability to facilitate specific product threads and its innovative audio feature. We'll end the conversation by looking at the future of yield and data analytics, offering valuable advice for the industry's next steps. Plus, we'll explore the integration of data from various sources through API, discussing the impact on sustainability and innovation in the industry. Get ready to be inundated with a wealth of insights into the realm of semiconductor yield management.
Thanks for tuning in to "Advantest Talks Semi"!
If you enjoyed this episode, we'd love to hear from you! Please take a moment to leave a rating on Apple Podcast. Your feedback helps us improve and reach new listeners.
Don't forget to subscribe and share with your friends. We appreciate your support!
Welcome back to Advantest Talks Semi, where we delve into the intricacies of semiconductor technology. Today, we're zooming in on a key metric that's vital to the success of our industry: yield. Yield, in simple terms, is the percentage of functional chips produced in a semiconductor manufacturing process. And, ideally, if all the units passed during testing, the yield would be 100%. If, on the other hand, 10% of the parts failed during testing, then the yield would be 90%. But it's not just a number. It's a reflection of efficiency, quality and profitability. I'm Keith Schaub and we'll explore how cutting-edge technology and precision engineering come together to push the boundaries of yield in semiconductor manufacturing. I'm joined by John O'Donnell, CEO of yield HUB, and Carl Moore, yield management specialist from yieldH UB, to discuss their innovative approaches in semiconductor yield management. Without further ado, let's delve into today's topics. John, Carl, welcome to Advantest Talks Semi.
John:Thank you, Keith.
Keith:John, let's start at the beginning. Tell us how yield HUB got started and what drew you into the yield management field in the semiconductor industry.
John:Well, thank you, Keith. So, I was always interested in data. I started as a test engineer in a large multinational and I was involved fairly early on with products that had big problems with yield, and over the years, I ended up being responsible for the yield management for that company and I went out to see what was available in the market for yield management back in the early 2000s. I wasn't that impressed at the time and I decided I could come up with something that would be more applicable to my company at the time, and then I realized that the ideas about it were generically relevant to the industry. I left that company in 2005 and started what was called MFG Vision, which has since been changed to yieldHUB. So, the mission was to help product and test engineers to easily analyze what was going on in test manufacturing. That's not an easy task because of the complexity of the data, the size of the data, the variability of the data and the intrinsic dirtiness of the data. The data isn't clean natively, so, big challenge, real insight, I felt at the time we had, was that to have a server-side solution where the software was not hosted by the user. It was a centralized database so that engineers could analyze in what, at the time, was probably not the cloud as their on-prem server, but that evolved into a cloud-based solution for the company called yield HUB.
John:Yield, as we know, is fundamental to the profitability of any company, and we believed we could bring small companies and larger companies more quickly into profitability for new products. Nowadays, the scalability of our original design is a big differentiator. The culture of the company is about collaboration. Our yield HUB platform is built on collaboration. We have also a diverse team over 40% women worldwide and we have over half of our actual engineers are in Asia. So, we have a good mix of engineers. We have a mix of data scientists. We have IT experts, program managers, technicians. We use best in class software for everything we do. Not only do we believe we're best in class in yield management, but we use third-party tools to help us manage the company really well as well.
Keith:Thank you, John. You mentioned something there about historical data used to be small or, as now, we have big data and big data has become mainstream in, I think, back in the early 2010s and, as you said, it's fundamentally transformed data analytics and how the industry approaches production efficiency and quality control. What is data analytics today and how does yieldHUB leverage big data and data analytics to drive improvements in semiconductor yield?
John:Big data today we see tens of thousands of tests with some customers, that's individual tests per chip. We see millions of units by thousands of wafers per quarter, so they generate terabytes of data per quarter. That, to us, is big data and it could be across hundreds of products.
John:Data analytics in case of big data, first of all, key thing is getting the data into a database. How do you process that amount of data? Some customers have tens of thousands per day. How do you process that into a database and technology to do that, number one. Number two, how do you actually analyze, say, thousands of wafers in a few seconds, because this is what these customers need to be able to do. So, the analytics required the design of the database really to allow that kind of analytics is really important.
John:Another key part of data analytics is these companies have multiple teams in different parts of the world. They need to work together. Hence, any solution for data analytics that is the most effective way to do it is to help teams collaborate and hence for example, stack 1000 wafers share what your observations with a colleague in Asia or in North America somewhere. Be able to do that instantly and being able to add your knowledge to the equation and build up the knowledge base. So, another key part of analytics these days is to be able to not only analyze the data but also comment on it and keep those comments in a thread for use by other people in your team who could be anywhere in the world.
John:The challenge of big data is being able to, first of all, process the data, be able to analyze quickly thousands and thousands of wafers and tests, and then be able to efficiently collaborate with your colleagues, because, at the end of the day, it's complex data. It's often beyond one person's skill set to really get to the core of an issue, so you need to be able to collaborate. That's what we really bring to the party.
Carl:Yeah, and another thing to consider there is just basically having all the data when you're looking at it. You're looking at a huge amount of historical data as well as the lots that you're looking at from a design point of view and also from a yield point of view that recently run. So, having all that data together, where you can combine that and look at it, really makes a big difference.
Keith:What kind of financial impacts does it have?
John:One example is that a customer recently told us from the States that by being able to analyze just hundreds of wafers and lots, they realized changing a test limit could save them hundreds of thousands of dollars a year. And that was just for one product. And this company would have hundreds of products, but this would have been a high-volume product. So it's amazing that a 1% yield improvement in a high-volume product can actually mean hundreds of thousands, if not a million of dollars in savings per annum. If you're producing 100 lots and you increase your yield by 1%, just 1%, you do not have to produce an extra lot every 100 lots, so that can be a significant amount of money. In most cases, companies will increase their yield using a system like yieldHUB by 2% or 3% over maybe a couple of years for key products. So there's millions of dollars in savings.
Carl:Yeah, and I think the other thing now to really look at is the fact that on the new nodes that these companies are using for some of the phones out there and some of the new high-tech stuff, the cost of wafers has just continued to go up higher. Even now, it's so much more important for that percent of yield to be saved.
Keith:Obviously, it's a huge opportunity if we can improve the yield even a fraction of a percent. What has been your experience with all these new data sources and formats and a lot of which are custom, because a lot of these companies build their platforms internally and how does yieldHUB manage the complexities and connect in to them and all these customizations.
John:Although there can be a wide variety of, say, flavors of a format. Our solution really is practical in that if you have an Advantest tester, if you have many product lines, it's very likely that there will be a slight variation in how the metadata is stored. For example, the lot-ID may have a slightly different format, or something. We make it very easy for customers to configure the parsers. They don't have to come to us, so it saves them a lot of money. The advantage here, of course, is that the data is much cleaner, and it's all about clean data to make really good decisions.
Carl:Yeah, we see a lot of different formats, and I think that just understanding what these customers are trying to do sometimes, especially with startups that might have a kind of a non-standard format. Because a lot of our staff is industry veterans, we've been able to go in and help them, really try to help standardize a little bit of what they're doing. But certainly, the flexibility of what we can do in the parsing and the fact that we understand what people are using the data from can really help in the whole overall solution.
Keith:Data analytics has been around for 30 plus years, but AI and machine learning - that's relatively new, and especially in production semiconductor testing. Do you see AI and ML as new challenges in the integration, and how does yieldHUB address that?
John:Yes, so our latest developments include AI and machine learning, and it is already making an impact. For any of this to be really effective, you know, as I was saying before about the cleanliness of data, we make it easy for customers to make the data clean. So the first step is clean data. Then the next step is linking the data. For example, linking the data from fab to wafer probe, wafer start to final test or to modules. Once you have all the data linked and clean, that's where you can make great effect from AI. So, for example, prediction. So, we have tools that predict eventual yield of a wafer using earlier data, from WAT data and wafer sort, and also tools for predicting reliability, so not just yield, reliability and efficiency. Also, we use machine learning and AI for some image analysis processing for certain industries. We see it as being very, very important. But also, AI cannot replace the human element. As I was saying before, the teams working together. And an engineer working with AI is really where we believe the real power is.
Carl:Yeah, I think, John, that's a great point because, really, when you look at some of the engineers we're working with, there's a lot of knowledge that these guys have and just tying that in with the AI and tying that in with the overall database is just super powerful.
Keith:So, I want to drill down a little bit on clean data versus dirty data. You hear these terms a lot in the industry. Walk me through, like, what is dirty data and what is clean data, and how do you get it from dirty to clean?
Carl:People get data from testers and often there's data in there that might be some rejects they don't want, or they run first pass of the data and then they have retest one and maybe retest two, and sometimes, you know, even up to three or four retests of the data. So, you have all this data with different yields. One of the important things in really processing that together to get clean data is to get a final yield for that product. So we take first yields and then the retest yields and combine those so that we get a final number and look at different things that might be outliers or counts that are missing. Sometimes you have units that might not have gotten a retest or extra units that have a retest and I think when you put all that together you really want accurate data because when you first look at a first pass of yields you might think "whoa, that's 60% yield, but ultimately, because of some retests that were done, it's really 95% and I think really just understanding those variations and pulling it all together to make it clean is important.
Keith:Oh, that's great, Carl. And so, once the data is cleaned, and, John, to go back to what you were saying, what are the benefits that you're seeing with combining AI and machine learning with data to get actionable insights?
John:Keith, thanks for the question. The key breakthroughs are in prediction, in predicting low yield, predicting outliers, and the advantage of being able to predict is that the material may be discontinued in the manufacturing flow and replaced by better wafers. Secondly, for reliability so been able to predict if units, individual units, will actually be reliable, and that's very important. And there are other applications we're rolling out for in the area of image analysis. So, a very strong suit of yieldHUB is image analysis for, say, CMOS image sensors and for other applications like that. We can detect and learn from defects that are detected in images and this helps automate processes that up to now have been done by human operators. So we're seeing very powerful applications in that area as well.
Carl:I think a lot of those points were really good. And then, some of the other areas that you get into is really looking at sometimes you have different, like in the image analysis, pattern recognition and trying to really help the engineer get through to find the solution. So, typically in the past you'd have the engineer trying to look at all the data and figure it out. So, if we can help guide them with a little bit of knowledge up front, that helps to get to the solution faster.
Keith:Perfect and that sort of gets into the global scalability. Many companies are also enhancing their data analytics capabilities by adopting more cloud- based solutions. They offer things like easier accessibility, better scalability and global resource sharing. What are some of the challenges and complexities, and how does yieldHUB address them?
John:Regarding the challenges of using the cloud. Well, it's kind of the opposite. We find that the cloud is actually ideal for the management of yield globally for companies. Sometimes people argue with, what about security in that? But the actual security nowadays in best in class cloud providers like we use is second to none and probably a lot safer than using on premise with multi factor authentication and secure transmission of data to one place. So, we see only advantages. Also sustainability, the ambitions of companies to be as sustainable as possible. The cloud is much greener, we believe, than on prem because the technologies in data centers are evolving and getting greener. We see the big advantages. We also see it helping companies in the sustainability efforts.
Carl:And, I think, the huge advantage is, the data is in one place. We're not moving it around in all the place. It can be accessed from anywhere. With a lot of these companies have engineers all over the world so the cloud helps to bring them together and really make things easy to just analyze and not worry about all the different pieces the day that you might have to do without the cloud and yieldHUB's been in the cloud for many, many years, so we've done a lot of work to optimize that throughout those years.
Keith:One of the major challenges in the industry, as you said, is power consumption, especially in the high- performance compute, and all the AI chips are bleeding edge and they consume a tremendous amount of power. How does yield management and yieldHUB help make the semiconductor manufacturing more sustainable?
John:One is, if you do improve your yield, you have to manufacture less, there fore energy saved. Energy in semiconductor manufacturing is significant. So, by having higher yield, you manufacture less units. The second one is the efficiency of your equipment. So, by monitoring things like idle time etc. you're using your equipment, your test equipment etc. more efficiently and there's less consumption of energy. Thirdly, with prediction, being able to predict wafers that are material that is not of sufficient quality that means that you're not manufacturing poor material, you stop manufacturing it early. Finally, with reliability, making more reliable components, which means that they last longer, so that they won't die after a couple of years and you have to manufacture more. So, you are actually reducing the manufacturing.
Carl:I just have to add a fifth one to that. That we can't forget is that yieldHUB can help with less engineering stress, and I think that's good for the environment as well.
Keith:So, we can live longer because of yieldHUB. That's great. So, Carl, going back to what you said with global collaboration, I'd like to understand that a little bit better. How does yieldHUB facilitate the global collaboration that is critical to today's success?
Carl:I think there's a couple areas. First of all, with engineers around the world, yieldHUB has the ability to share things quickly to put in comments too. You can even record audio to add comments that people can listen to, and I think that the ability to do that and send a report the receiver can start working on right away and collaborate between these people across the world is important. The other thing is when you look at collaboration between, say, product engineers, test engineers, designers, data scientists, they can all because they're working off the same system, it's easy for them to look at things together and not have to figure it out with all the different formats. So, that's really powerful to pull that together and allow them to do that.
John:So, traditionally, systems in a big company, for example, you may have multiple systems because engineers, say, in California might be using a different data analysis system to somebody in Manila or Vietnam or whatever.
John:With a platform that's as easy to learn as yieldHUB, the situation we find is, what's happening is, that people aren't throwing data over the wall to somebody else to continue on to analyze in their own systems. They're using the common platform. What you're doing is you're sharing the analysis and reporting live. You're not sending raw data and then somebody has to spend another couple of weeks figuring out and trying to agree with you over something. That is actually a really tangible effect of having a platform, an online platform like yieldHUB. So, it really does enhance collaboration across the world, and that's something that's part of our vision is that the engineers in the manufacturing facilities, which could be anywhere in the world, are actually on the same page very, very quickly with the design part of the company or the R&D side. The issues are quickly resolved because of really good teamwork. So, we're encouraging our customers in the way we roll out our systems, to talk to each other and be there for each other on the platform.
Keith:Because of the platform approach and the ease of use, you get this democratization analysis where you bring in more people that are able to actually analyze and help resolve problems much faster.
John:Yeah, exactly, and it basically aims to eliminate the silos of information. You're encouraging people to work together and they can see the benefits of sharing the knowledge they have and the expertise online. Because, again, key dimension is that you can look at some situation, you can write down or you can save your voice, you can save audio and associate with issues and then the person on the other side of the world, wherever that is, can listen to you if they can understand your accent, but they will read it anyway. But the knowledge is spread. The way of fixing the root cause is going to be accelerated.
Carl:Yeah, I don't think I have too much of an accent, John, but we see a lot more design and there's actually starting to tools like this because they want to upfront, make their design powerful and work with the people. So, we just see a lot more of that starting to happen.
Keith:John, Carl, you mentioned the audio feature, which is a very unique feature. I hadn't thought about that. I didn't know that was part of the yieldHUB solution. So, building on that a bit, what are some of the other unique features that yieldHUB has that sets it apart from other tools that you see in the market today?
John:So, Keith, for example, if you have a tester, say an Advantest tester and you know that that's a really good tester.
John:What you can do is you can follow that tester, so like following the tester on Twitter, right. So, you follow it on X and if anybody complains about the tester or says something about it behaving unusually about a product, you will be notified and you will be able to add your knowledge and say, actually, well, you know you need to set up the testers slightly differently or you need to do this, and that this is very unique, I believe. So, it's not so much even yield issues, but the equipment. You can be the expert for equipment, like testers, and you can actually have your own thread, like in Facebook or LinkedIn or whatever a thread on a tester. It could be on a product, it could be in a test program, it could be on a subcon, it could be on anything meta, right? So, basically, you can set up these threads and other people can follow the threads and also contribute to the actual knowledge. So, we believe that's very unique.
Keith:That is super unique. I've never heard of that or thought about that, but now that you describe it, being able to follow a tester, I mean the concept is, I would never have thought of that, but the benefits are obvious once you explain it. That's very interesting.
John:So, you could follow a tester, you could follow a tester type, you could follow a handler.
Keith:Can you give it a "thumbs up?
John:I find that you can and we find the advantage of the obviously a lot of thumbs up. Actually, you can. Yeah, you can put everything you want, emojis are in there as well.
Carl:Yeah, another thing too, if you carry a phone, you can access yieldHUB from your pocket. So if you're out at lunch you can actually be looking at yieldHUB, get the alerts, which is great. And that thing you're following pops up. You'll see it right on your phone, but not just the text message or whatever, but you can actually call up yieldHUB and you know your screens right there and you can do a full analysis because of the web- based features of it.
Keith:What's your take on where all of this is going and what is your advice? What should we be thinking about in the near future in regards to yield or data analytics or yieldHUB?
John:We're also working on the edge and that will be important, and I believe this good work from Advantest, that here as well. But we believe that you can delegate some of the cloud stuff onto the tester and the edge and, in fact, can you believe it - that I set up the company originally with that in mind? Real time, so we have that real time capability. So, we believe the future will be a combination of the central server working on the edge as well, working with edge software. Also, we believe this net zero and sustainability would become really, really important. So, efficiency test time is important and a simple thing is not leaving testers switched on over the weekend, if not using them. So, helping that, the intelligence of the visualization of helping people test more efficiently and using testers that are efficient, integrating multiple sources of data to help with that, for example, integrating financial information with the yield information, with the efficiency information - all that so that you can really prioritize. So, just bring in more sources of data and metadata is going to be important.
John:Obviously, prediction and AI working with experts is really important. Our new products are all about opening up the system to other systems via API. So, for example, a wafer map, which may have out there detection from yieldHUB, would be fed into another system which would actually have some other proprietary algorithm that may include data from some we can't see. But it can update then the wafer map, you can feed it back into our platform and out into the official map for that wafer. So, opening up our system for that kind of uses it's all right. We already do it but in the next generation is really part of the core system.
Carl:So, it's basically the combination of being much faster, leveraging the edge, having many, many sources of data to help with sustainability as well is, I think, gonna be very, very important. JOHN (guest): I think the other areas, the data just continues to get bigger and I think the ability to analyze that is important, and even nowadays, you're looking at some of these new areas with chiplets where they're combining many different things into one so that it just becomes again much, much bigger in terms of the amount of data that gets output by the tester, and I think we have to be able to do something with that data and figure out what that data means.
Keith:So, John, Carl, thank you for coming on to Advantest Talk Semi and hope to see you at the next conference.
John:CARL (guest) Thank you, Keith, it's been a pleasure! JOHN (guest): Thanks for having us, Keith.